2 Uncovering transdiagnostic influences on experiential and observational learning: a study plan
2.1 Introduction
Decision-making in humans is guided by learning, both from personal experience, and the actions and choices of others (Lee et al., 2012; O’Doherty et al., 2017; Sutton & Barto, 2018). The former reflects experiential learning (EL) (O’Doherty et al., 2004), where actions that were previously rewarded are more likely to be re-selected, while actions previously punished are more likely to be avoided. Conversely, observational learning (OL) (Joiner et al., 2017) is vicarious, by which agents are able to determine the rewarding or punishing outcomes associated with specific actions without the need to personally engage in exploratory or risky behaviour (Dunne & O’Doherty, 2013). To this end, agents learn to imitate or develop a causal understanding of others’ successful actions (Cushman, 2024; Suzuki & O’Doherty, 2020), by employing several strategies including the capacity to learn from the rewards experienced by others, and from inferring their goals/intentions (Charpentier & O’Doherty, 2018; O’Doherty et al., 2021).
In certain social environments, humans may integrate both OL and EL in the decision-making process (Charpentier et al., 2023; Pärnamets & Olsson, 2020; Zhang & Gläscher, 2020), by tracking signals associated with direct experience and vicarious valuation with a combined reward/social prediction error (Zhang & Gläscher, 2020). However, there is considerable heterogeneity across individuals who may also switch between EL and OL according to the reliability of each strategy (O’Doherty et al., 2021), or evoke a single strategy of EL-only or OL-only (Charpentier et al., 2023). Ultimately, OL and EL, either collectively, sequentially, or separately, are integral processes when making informed social decisions in complex environments.
Computational frameworks also provide a formal account of behavioural changes observed in mental health disorders (Huys et al., 2016, 2021), where model parameters can be objectively compared across cohorts. Recent literature has shown learning biases across a range of psychopathologies, including difficulties with learning from uncertainty (Aylward et al., 2019; Browning et al., 2015) and punishment (Pike & Robinson, 2022) among those with mood and anxiety disorders, whilst several studies have demonstrated an association between anhedonia, the core symptom of depression, with reduced learning from rewarding outcomes (Admon & Pizzagalli, 2015; Eshel & Roiser, 2010). Furthermore, individuals with social anxiety demonstrate biased learning from socially-specific information (Beltzer et al., 2019; Piray et al., 2019), including positive and negative social feedback (Button et al., 2012; Koban et al., 2017, 2023; Zabag et al., 2022, 2023), and are more accurate at learning to avoid social punishment (Abraham & Hermann, 2015; Voegler et al., 2019). These learning biases lead to the behavioural symptoms observed in social anxiety, including the maintenance of a negative self-view and low self-esteem (Koban et al., 2017), perception of low social rank (Gilboa-Schechtman et al., 2017) and the continued avoidance of social situations (Peterburs et al., 2022).
However, conventional RL paradigms used to assess social learning do not accurately represent complex social environments, where agents may integrate multiple learning strategies in the decision-making process. To this end, neuroeconomic games present an ecologically valid approach for assessing social behaviour by replicating real-world social interactions in a controlled setting (Glimcher, 2014; Loewenstein et al., 2008), a methodology highly recommended for use in behavioural and psychiatric studies due to its wide applicability (Hasler, 2012; Kishida et al., 2010; Montague, 2018; Robson et al., 2020). In such games, individuals with social anxiety are more likely to conform to others’ behaviour or judgements (Bică et al., 2021; Feng et al., 2018), reflecting an excessive need for social approval (Leary et al., 1988). Similarly, clinically depressed individuals are more susceptible to informational social influence (Hofheinz et al., 2017), influenced by self-esteem, a negative predictor of social anxiety (Fatima & Ghayas, 2017; Seema & Kumar, 2017). Individuals with low trait self-esteem are also more heavily influenced by others when making social decisions (Lee & Chung, 2022), suggesting that socially anxious individuals demonstrate a bias towards observational learning.
A major goal of psychological research revolves around associating changes in task performance with behavioural traits or measurements. Yet, measures of psychopathology are conventionally reduced to a single dimension or score, despite high-levels of heterogeneity observed among psychiatric disorders (Feczko et al., 2019), including social anxiety disorder (SAD) (Spokas & Cardaciotto, 2014) and depression (Fried, 2017), reflecting a complex behavioural profile not captured by a single dimension or questionnaire. A transdiagnostic approach to psychopathology (Gillan et al., 2016; Robbins et al., 2012) conversely favours a continuous definition of mental illness, present among normal variations in psychopathology in the general population. By employing data-driven dimensionality reduction on questionnaire batteries assessing a wide-berth of psychopathology, latent factors of mental health can be extracted that more accurately map onto candidate learning processes than any individual questionnaire score (Gillan & Seow, 2020). Computational factor modeling (CFM) (Wise et al., 2023) further combines a transdiagnostic approach with theory-driven computational modeling of behaviour, to infer the computational processes that characterize a given factor. To date, CFM has identified transdiagnostic associations with model-based planning (Gillan et al., 2016), metacognition (Rouault et al., 2018), reward processing (Suzuki et al., 2021) and uncertainty (Norbury et al., 2018), offering an objective formal approach for demonstrating learning biases at the transdiagnostic level.
To date, a single study has applied a transdiagnostic approach to EL and OL, finding that heterogeneity in strategy use is characterized along the two psychiatric symptom dimensions of autistic traits and trait anxiety (Charpentier et al., 2023). Surprisingly, there were no significant influence of the transdiagnostic dimensions of social anxiety or social dysfunction. This may be a consequence of the task design, which only involved observation of a single other simulated agent, differing from complex social environments where agents must learn from multiple others. Furthermore, the study did not measure the behavioural effects of confidence, which influences choice (Campbell-Meiklejohn et al., 2017; De Martino et al., 2013) and is negatively correlated with self-reported depression, social anxiety, and generalized anxiety scores (Rouault et al., 2018). In addition, the questionnaire battery consisting of only four questionnaires excludes certain components of psychopathology, with the latent factor structure unvalidated. A more comprehensive, validated battery may therefore be more suitable for extracting latent factors of psychopathology.
In our planned study, we aim to identify the transdiagnostic symptom dimensions underlying OL and EL by recruiting a large general population sample, tasked with completing a social influence task in groups. We will dissect the specific contributions of OL and EL to the decision-making process through computational modeling, identifying heterogeneity in strategy use across the whole group, and across transdiagnostic factors. By taking an objective, computational approach, we aim to identify candidate learning processes that can be targeted through pharmacological and psychotherapeutic interventions.
2.2 Methods
2.2.1 Participants
Participants will be recruited from the online platform Prolific, with only those between the ages of 18-50, a completion rate of 95% and with more than 20 completed studies eligible. We aim to recruit 500 participants, similar to previous studies (Hoven et al., 2023; Rouault et al., 2018; Seow & Gillan, 2020) demonstrating a strong replicability of the original factor loadings reported in a larger sample (Gillan et al., 2016). Given the average exclusion rate of approximately 15% for online behavioural studies (Chandler et al., 2014) we aim to initially recruit 600 participants. Exclusion criteria will include a history or current diagnosis of neurological/psychiatric disease or current medication. To avoid gender bias, each group will consist of only same-gender participants.
2.2.2 Assessments
Participants will firstly complete a questionnaire battery (Gillan et al., 2016), reliably assessing a wide berth of psychopathology in a general population sample. Specifically, we will run a reduced sample of 71 questions reliably found to replicate the same 3-factor structure, to increase participant retention (Hopkins et al., 2022). The questionnaire battery will assess obsessive-compulsive disorder (OCD) using the Obsessive-Compulsive Inventory – Revised (OCI-R) (Foa et al., 2002), depression using the Self-Rating Depression Scale (SDS) (Zung, 1965) trait anxiety using the trait portion of the State-Trait Anxiety Inventory (STAI) (Spielberger, 1970), apathy using the Apathy Evaluation Scale (AES) (Marin et al., 1991), eating disorders using the Eating Attitudes Test (EAT-26) (Garner et al., 1982), impulsivity using the Barratt Impulsivity Scale (BIS-10) (Patton et al., 1995), and social anxiety using the Liebowitz Social Anxiety Scale (LSAS) (Liebowitz, 1987). IQ will also be measured as a co-variate using the Wechsler Adult Intelligence Scale (WAIS) (Wechsler, 2019).
2.2.3 Task structure
After completing the questionnaires on Prolific, participants will be invited in groups of five later the same day to complete a social influence task (Zhang & Gläscher, 2020), a multistage group decision-making paradigm enabling participants to learn directly from their own experience and vicariously from observing others. The task will be implemented using oTree (Chen et al., 2016), an open-source platform with the capacity to real-time multi-player experiments online. The basic experimental setup consists of an experiment written within oTree, a server computer, which can be a cloud server or a local laptop, and subjects’ devices with a web browser to access the experiment (Fig 2.1). We selected oTree over alternative platforms including LIONESS Lab (Giamattei et al., 2020) and nodeGame (Balietti, 2017), due to its minimalistic and robust framework, as well as its popularity (>1900 citations as of May 2024).
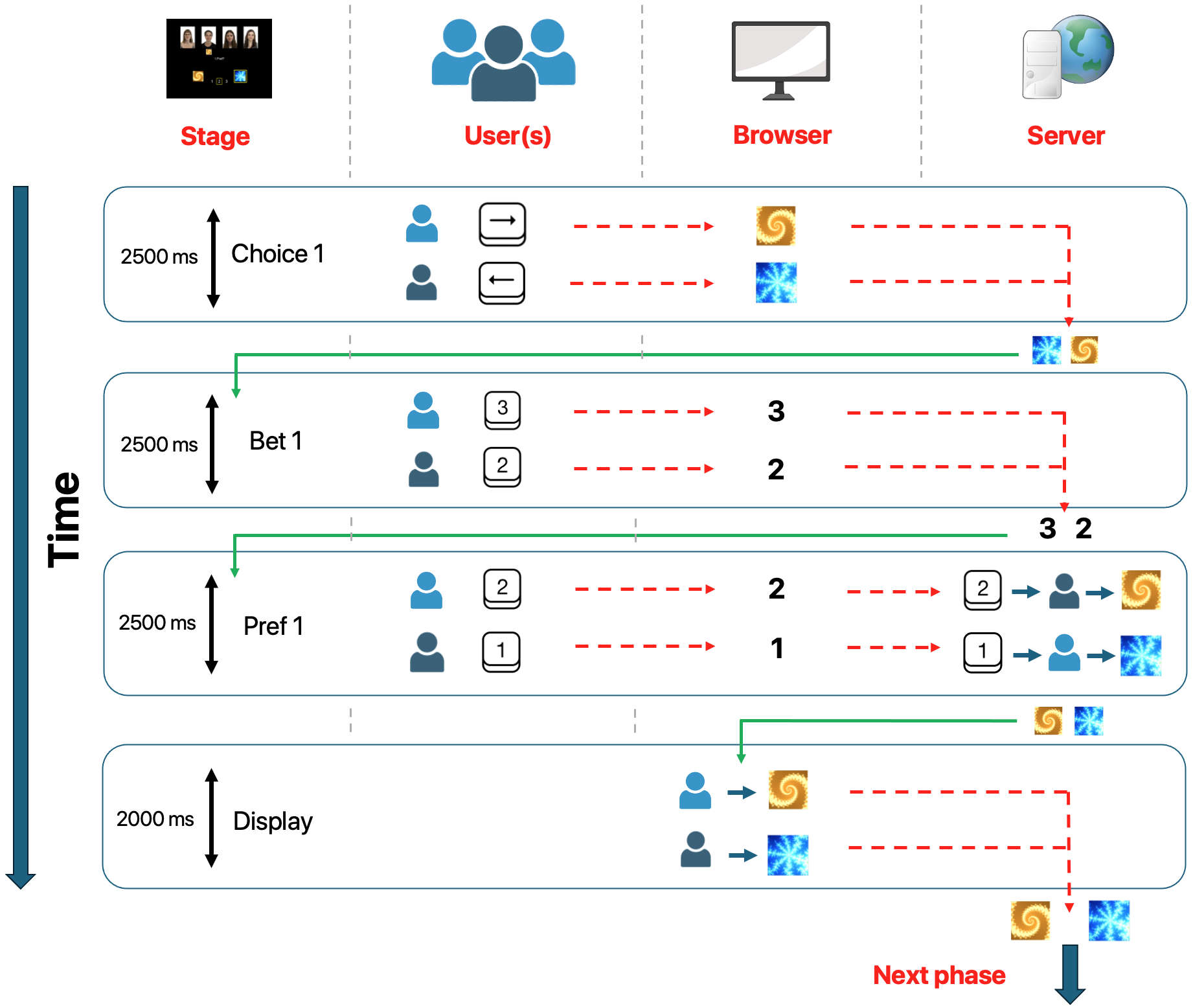
Figure 2.1: The oTree framework for a single page of the social influence task. When beginning the task, the player firstly enters their initial choice of the two fractals by pressing either the left or right arrow key. At the time of the button press, this response is both logged by the browser locally, and on the server. Subsequently, the end of the Choice 1 phase (determined by the end of a 2500ms interval) triggers the Bet 1 phase, where players must make their bet by pressing either the ‘1’, ‘2’ or ‘3’ button. Players’ button presses are similarly logged by the browser and sent to the server at the time of the bet being made. Players are moved to the preference choice phase at the end of the allotted time. In the preference choice phase, players must select another participant within the group whose choice they will uncover. The button press is similarly logged, corresponding to the number assigned to the other players within the group. The server then retrieves the image selected by the player chosen in the choice phase. These images are then displayed, after which players are moved on to the next page consisting of the second choice, second bet and trial feedback. The page structure depicted reflects a simplified version of the actual task.
Upon joining a session, participants will be given detailed instructions regarding the task, after which they will individually play a practice session of 10 trials against four computerized opponents. They will then be placed into a waiting room for the other four players to arrive at the same point. The waiting room will include a ‘live chat’ function, both to reduce participant dropout and to demonstrate the legitimacy of the experiment. Once all five players have entered the chat room, after 30 seconds they will be transferred to the main experiment.
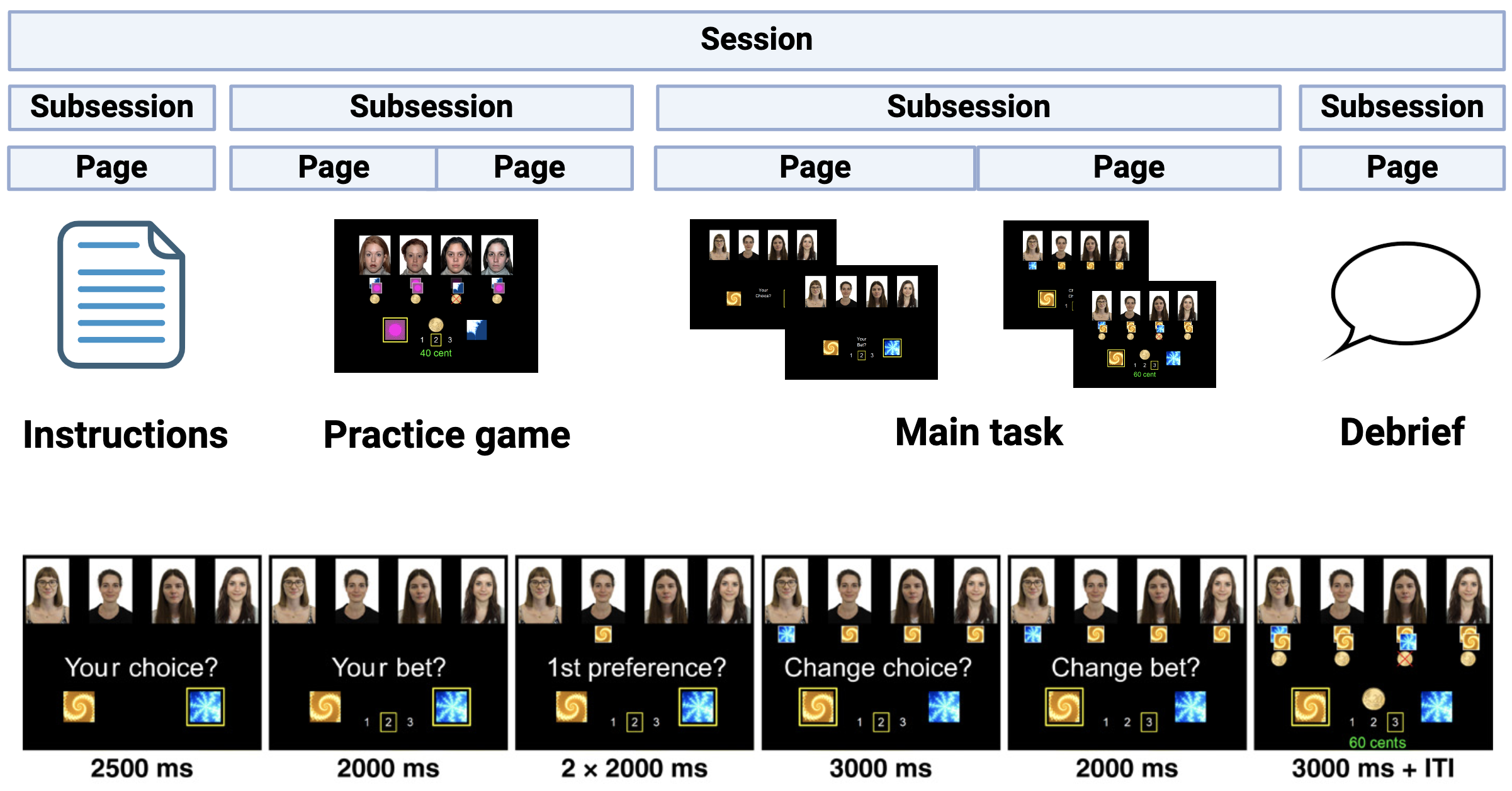
Figure 2.2: The general structure of the experiment under the oTree framework. Each ‘Session’ consists of several ‘Subsessions’ which constitute a distinct component of the experiment. Each ‘Subsession’ itself consists of ‘Pages’ representing each stage of the task. The main social influence task consists of two main pages with page one containing the Choice 1, Bet 1 and Preference phases, whilst page two contains the Choice 2, Bet 2 and trial feedback. Each of the six stages are visually depicted along with the allotted time. Certain components of the figure adapted with permission from (Zhang & Gläscher, 2020).
2.3 Planned analyses and results
2.3.1 Factor analysis
Replicating a popular approach implemented in previous studies (Gillan et al., 2016; Hoven et al., 2023; Rouault et al., 2018; Seow & Gillan, 2020), we aim to perform our factor analysis with Maximum Likelihood Estimation (MLE) using the Psych package in R, with an oblique rotation. We will subsequently select the number of factors based on Cattell’s criterion (Cattell, 1966), using the Cattell-Nelson-Gorsuch test (Gorsuch, 2014). Replicating previous research, we expect a three-factor model ‘Anxious-Depression’, ‘Compulsive Behaviour and Intrusive Thought’ and ‘Social Withdrawal’ to best describe the data structure. Our factor analysis will be validated by running Pearson’s correlations between item loadings obtained from the factor analysis in (Hopkins et al., 2022) using the same 71 items (Fig 2.3).
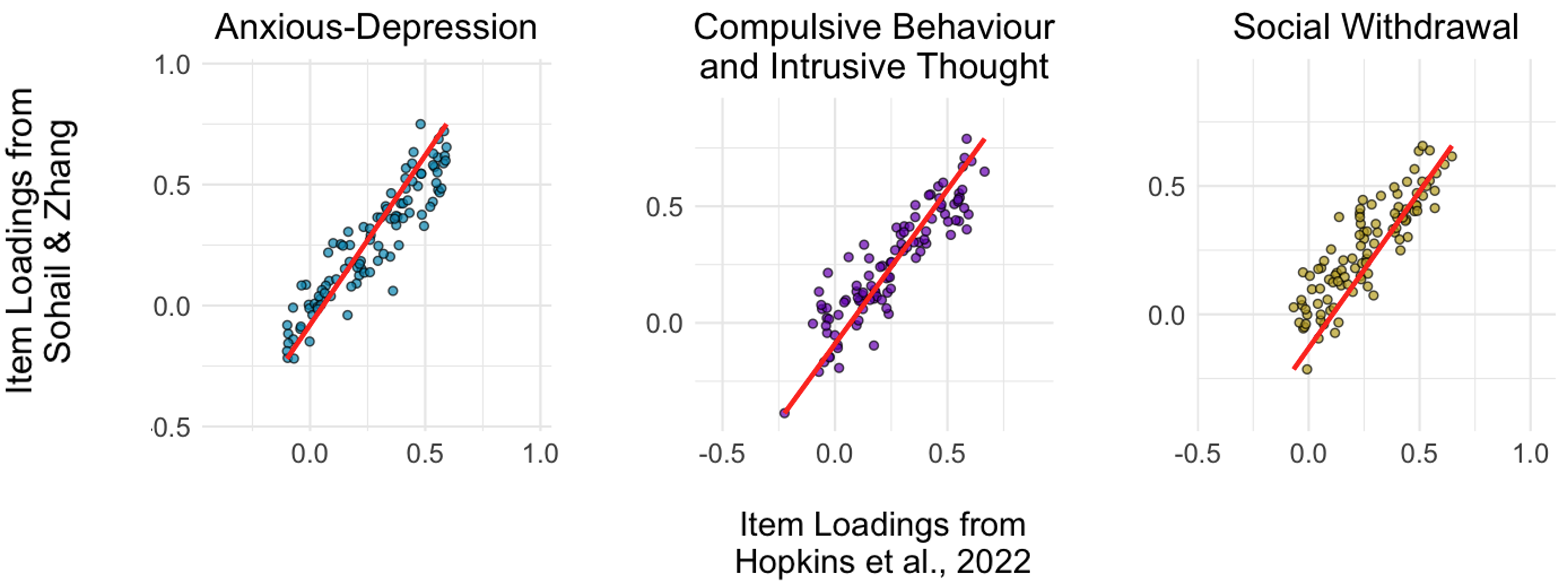
Figure 2.3: Predicted correlations between item questionnaire loadings for the study and (Hopkins et al., 2022).
2.3.2 Behavioural analysis
Across the whole cohort, we look to replicate the behavioural results reported by (Zhang & Gläscher, 2020), who also ran the same paradigm in a healthy population sample. Namely that:
Participants will show an increasing trend to switch their choice toward the group when faced with more dissenting social information, and are more likely to persist when observing agreement with the group.
Participants will tend to increase their bets as a function of the group consensus when observing confirming opinions but sustain their bets when being contradicted by the group.
Participants’ choice accuracy of the second decision will be significantly higher than that of the first, and participants’ second bet will be significantly larger than their first.
To determine the transdiagnostic effects upon these behavioural measures, we plan to run moderation analyses for each factor separately. Reflecting higher levels of social influence on choice through lowered confidence and higher social approval, we expect to see the following associations with both the ‘Social Withdrawal’ and ‘Anxious-Depression’ transdiagnostic factors:
An increased tendency to conform to group choices when observing agreement.
A reduced tendency to increase their bets when observing confirming opinions, and increased tendency to lower their bets when being contradicted.
Participants’ choice accuracy of the second decision will be significantly higher than that of the first, whilst participants’ second bet will not significantly differ from the first.
2.3.3 Computational modeling
To uncover the distinct influence of OL and EL on social decision-making, we will perform trial-by-trial modeling under the hierarchical Bayesian framework using RStan. We will use the same range of non-social and social computational models as a previous study implementing the same paradigm (Zhang & Gläscher, 2020). These firstly include baseline models which did not consider any social information (category 1: M1a, M1b, and M1c). Instantaneous social influence (i.e., other players’ Choice 1, before outcomes were delivered) was then included on top of category 1 models, to construct the first category of social models (category 2: M2a, M2b, and M2c). And finally, social learning parameters were added, reflecting competing hypotheses of value update from observing others (category 3: M3, M4, M5, M6a, and M6b). However, instead of describing a singular ‘winning’ model explaining all participants’ data, we aim to implement a heterogeneous approach used to describe different strategies employed across the sample (Charpentier et al., 2023). To this end, we aim to fit the same models to each individual, calculating the winning frequency of each model across all participants (Fig 2.4). Model verification will be performed through a parameter recovery analysis to assure that all parameters can be accurately and selectively identified, and posterior predictive checks which should accurately capture behavioural patterns observed in the data.
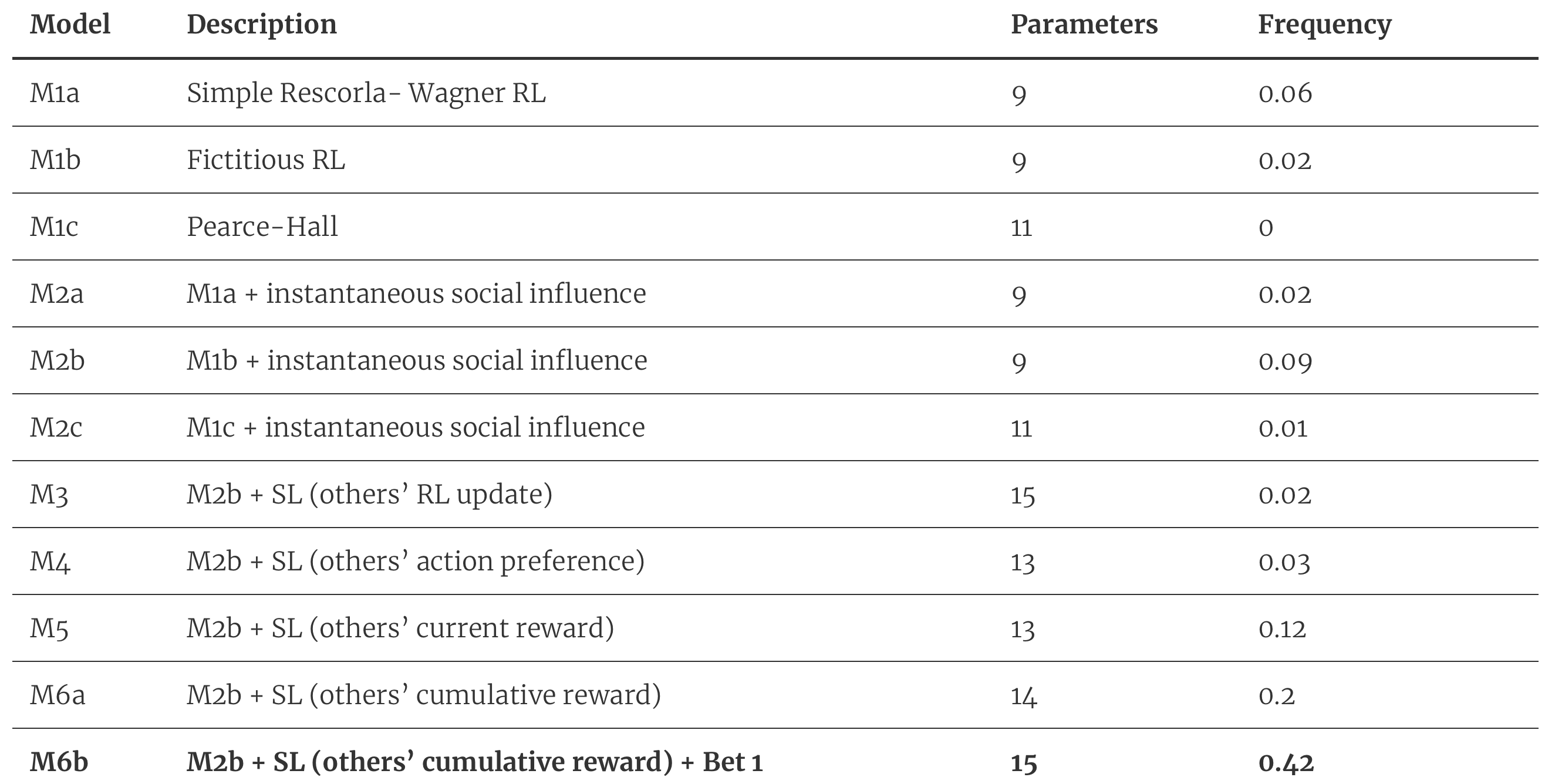
Figure 2.4: Candidate computational models with predicted model frequencies for the entire cohort. Candidate computational models with predicted model frequencies for the entire cohort. Our candidate models consist of both non-social and social models featuring different parameters. The model with the highest winning frequency (M6b) is highlighted in bold.
Replicating previous results, we expect for a combined social model (M6b) consisting of a fictitious RL with additional parameters representing instantaneous social influence, bet 1 and other’s cumulative reward, to constitute the winning model for the highest proportion of participants. This suggests that most participants jointly integrate value signals computed from direct learning and social learning to guide future decisions. Within the winning model (M6b), parameters reflecting self - Beta(Vself) - and other - Beta(Vother) - should be comparable, both predicting the accuracy of Choice 1. This reflects the integration of self and other-directed information under the combined model (Fig 2.5a). However, we expect for other models to also represent winning models, albeit in fewer participants, demonstrating the heterogeneity in strategy use across our sample (Fig 2.5c). Furthermore, within both M5 and M6a groups, we predict a significantly greater difference between parameters reflecting self - Beta(Vself) - and other - Beta(Vother) - with other-directed information demonstrating a stronger influence.
Across the whole group, we also expect to observe a strong positive relationship between the effect of dissenting social information - Beta(w.Nagainst) - and the susceptibility to social influence, and between the effect of confirming social information - Beta(w.Nwith) - and the extent of bet difference. This suggests that individuals are more likely to change their choice in response to group choice difference, and that confidence increases if a player’s choice is also chosen by the group. To determine the transdiagnostic influences upon choice and choice confidence in response to social information, we plan to run separate moderation analyses for each transdiagnostic factor upon the relationships between the effect of dissenting social information - Beta(w.Nagainst) - and the susceptibility to social influence, and the effect between confirming social information - Beta(w.Nwith) - and the extent of bet difference. We subsequently expect for both ‘Anxious-Depression’ and ‘Social Withdrawal’ factors to have a significantly positive moderating effect upon the former and a significantly negative moderating effect upon the latter, reflecting lowered confidence in one’s own choices (Fig 2.5b).
Finally, to assess whether the groups within our sample - split by the winning models - differ on the three transdiagnostic symptom dimensions, we will run a linear mixed model predicting the factor scores from an interaction between symptom dimension and group, including a random intercept, and controlling for gender, age, education, and IQ. Reflecting the tendency for those with increased impulsivity and compulsivity to disregard accumulated information, basing decisions on more recent outcomes (Franken et al., 2008; Kim & Lee, 2011; Robbins et al., 2012; Vaghi et al., 2017), we expect the M5 winning model group (fictitious RL + instantaneous social influence + others’ current reward) to feature significantly higher factor scores for ‘Compulsivity-Impulsivity’. On-the-other-hand, reflecting the ability to integrate historical social information - but not one’s own confidence - when making social decisions, we predict for the M6a winning model group (fictitious RL + instantaneous social influence + others’ cumulative reward) to feature significantly higher factor scores for ‘Anxious-Depression’ and ‘Social Withdrawal’ (Fig 2.5d).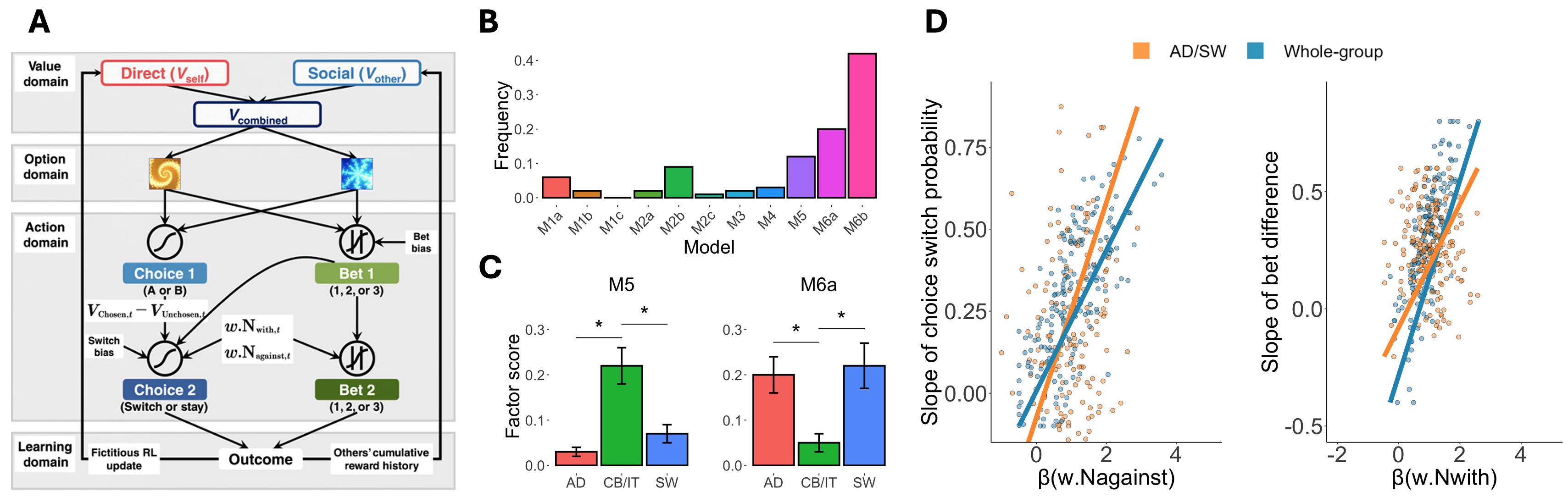
Figure 2.5: Transdiagnostic factors alter social learning strategies A) Schematic representation of the winning model (M6b) where participants’ initial behaviours were accounted for by value signals updated from direct and social learning and behavioural adjustments ascribed to initial valuation and preference-weighted instantaneous social information (Reproduced from (Zhang & Gläscher, 2020) with permission). B) Model frequencies across the whole cohort reveal a primary use of the M6b model, but also demonstrate heterogeneity in social learning. C) The winning model groups M5 and M6a feature significantly higher factor scores for ‘Compulsive Behaviour / Impulsive Thoughts’ and both ‘Anxious-Depression’ and ‘Social Withdrawal’ respectively. D) Visual depiction of the influence for both ‘Anxious-Depression’ and ‘Social Withdrawal’ factors upon choice and confidence, where the relationship between the effect of confirming social information - Beta(w.Nwith) - and the extent of bet difference weakens, and the relationship between the effect of dissenting social information - Beta(w.Nagainst) - and the susceptibility to social influence strengthens. Note that this is a visual representation of the moderation analyses, and will not be an actual analysis performed (i.e., a group-level comparison).